Fraunhofer IIS - The carbon footprint of edge AI applications results largely from the production of hardware components and especially batteries. Benchmarking energy consumption and latency per inference on microcontrollers enables resource-conscious design decisions to develop environmentally friendly and efficient AI applications. This tech blog describes the procedure of such an energy efficiency-driven development process using a concrete example.
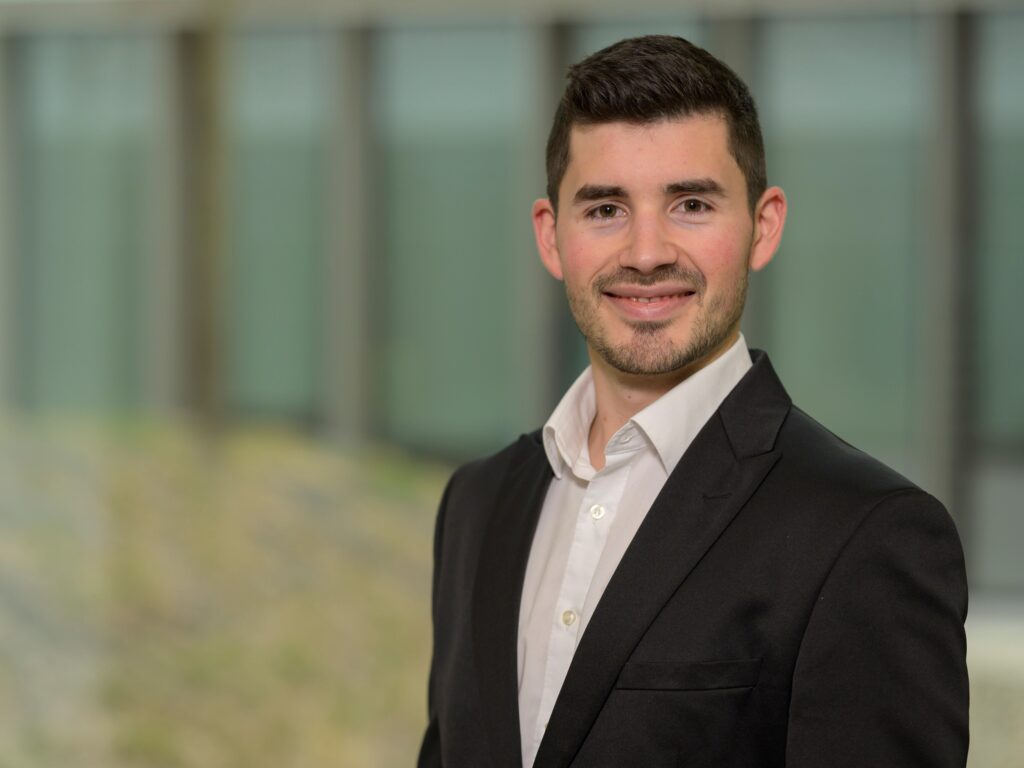
Edge AI hat das Potenzial, die CO2-Emissionen erheblich zu senken, indem es ermöglicht, Künstliche Intelligenz direkt auf lokalen, oft kompakten Geräten, den sogenannten “Edge Devices”, auszuführen. Diese Technologie findet Anwendung in vielen verschiedenen Bereichen. In der Landwirtschaft kann EdgeAI helfen den Gesundheitszustand von Pflanzen zu überwachen uns so dazu beitragen den Ertrag der Ernte zu erhöhen. Edge AI kann dazu beitragen, Biodiversität zu erhalten und Wilderei zu verhindern indem großflächig Naturgebieten überwacht werden. Es findet auch im Bausektor und in der Gebäudetechnik Anwendung und kann dazu beitragen den Energieaufwand zu reduzieren und Fehlverhalten in wichtigen Geräten frühzeitig zu erkennen [1]. Da Edge AI einen großen Beitrag zur globalen Nachhaltigkeit leisten kann, wird der eigene CO2-Fußabdruck dieser Technologie häufig übersehen – ein Aspekt, der bei der Entwicklung von Edge-AI-Anwendungen jedoch nicht außer Acht gelassen werden sollte.
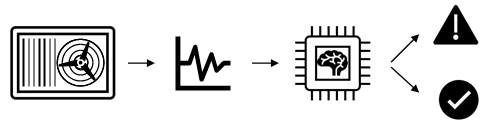
Diese Technologie findet Anwendung in vielen verschiedenen Bereichen. In der Landwirtschaft kann EdgeAI helfen den Gesundheitszustand von Pflanzen zu überwachen und so dazu beitragen den Ertrag der Ernte zu erhöhen. Edge AI kann dazu beitragen, Biodiversität zu erhalten und Wilderei zu verhindern indem großflächig Naturgebieten überwacht werden. Es findet auch im Bausektor und in der Gebäudetechnik Anwendung und kann dazu beitragen den Energieaufwand zu reduzieren und Fehlverhalten in wichtigen Geräten frühzeitig zu erkennen [1]. Da Edge AI einen großen Beitrag zur globalen Nachhaltigkeit leisten kann, wird der eigene CO2-Fußabdruck dieser Technologie häufig übersehen – ein Aspekt, der bei der Entwicklung von Edge-AI-Anwendungen jedoch nicht außer Acht gelassen werden sollte.
Here we use a specific example to show how data-supported, sustainable edge AI systems can be developed in a targeted manner. To this end, we would like to take a closer look at the development of a simple retro-fit system that uses vibration data to identify fault conditions in heat pumps. The device is designed to detect faults at an early stage and thus prevent people from being left in the cold unexpectedly. However, it should be noted that the application is simplified here and is only intended to illustrate how such a development process could work.
The carbon footprint of edge AI systems
A significant part of the carbon footprint of edge AI systems is generated during the production of the hardware, particularly by the batteries. Studies show that batteries have a carbon footprint that is 100 times higher than that of mains power [2, 3]. However, they are indispensable in many edge AI applications. The capacity of the battery is therefore an important resource that limits the maintenance-free service life of the entire system and should therefore be used as efficiently as possible. In our example, the system should last at least one year without a battery change, as heat pumps are serviced annually.
The aim is therefore to keep the average power consumption as low as possible so that the smallest possible battery can be used for a given maintenance-free service life, thereby reducing the system's carbon footprint. The choice of microcontroller therefore plays a decisive role in the development process. This must fulfill two essential requirements:
- Computing and storage capacity: It must be possible to execute the AI model.
- Energy-efficient standby mode: The lowest possible energy consumption in energy-saving mode.
AI model training
The size of the AI model used significantly influences the hardware requirements (RAM, ROM) as well as the computing effort, execution time and energy requirement per inference. A smaller AI model requires fewer hardware resources and generally also has a lower energy requirement per inference.
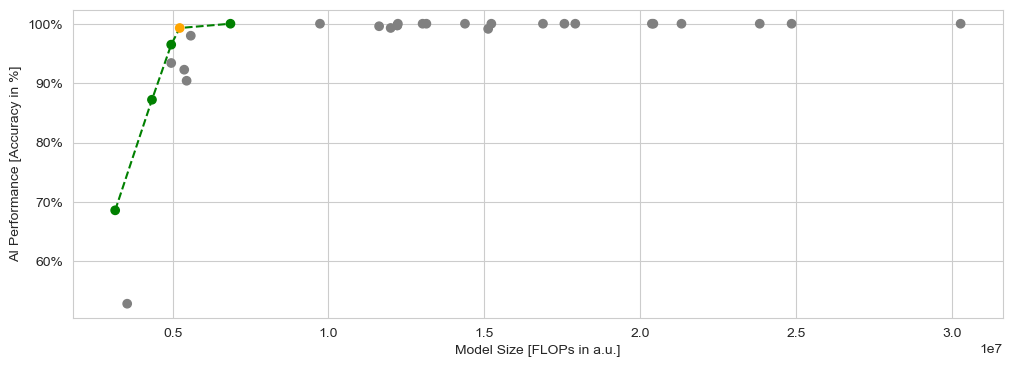
However, there is often a trade-off between model size and performance. We therefore use multi-objective optimization in the development of AI models to find an optimal compromise between model performance and model size. The following figure shows the result of this optimization. On the x-axis, the model size is represented by the number of required computational instructions per inference. The y-axis shows the model accuracy on the test data. Each point represents an AI model. Along the green line, the so-called Pareto front, are the models that have the highest accuracy for their size. For further investigation, we opt for the model marked in orange. This offers a good compromise between model size and AI performance, as it correctly identified almost all errors from the test data as such, but is still relatively small.
On-device energy benchmarking
The choice of AI model determines which microcontrollers come into question. In our example, we will limit ourselves to comparing two commercially available microcontrollers: the MIMXRT1062 and the nRF52840. The following table compares some key features, including the processor architecture, clock speed, power consumption in low power mode and the required operating voltage. Although the MIMXRT1062 is more powerful, it is less efficient than the nRF52840 in low power mode.
Microcontroller | Architecture | Clock rate [MHz] | Energy saving mode [mA] | Voltage [V] |
MIMXRT1062 | Arm Cortex M7 | 600 | 1,604 | 3.3 |
nRF52840 | Arm Cortex M4F | 64 | 0,301 | 3.3 |
To determine the energy consumption per inference, the AI model is executed on the microcontrollers while the current is measured at a constant voltage. Since a single inference is often too fast to measure precisely, we run several inferences in succession and calculate the average energy consumption and the average inference duration. For the nRF52840, 100 inferences and for the MIMXRT1062 1000 inferences were carried out in succession in order to achieve a suitable measurement duration. Half a second was waited between each of these “inference blocks” in order to be able to reliably identify the inferences in the current signal. The following graph shows the course of the current intensity during the measurement.
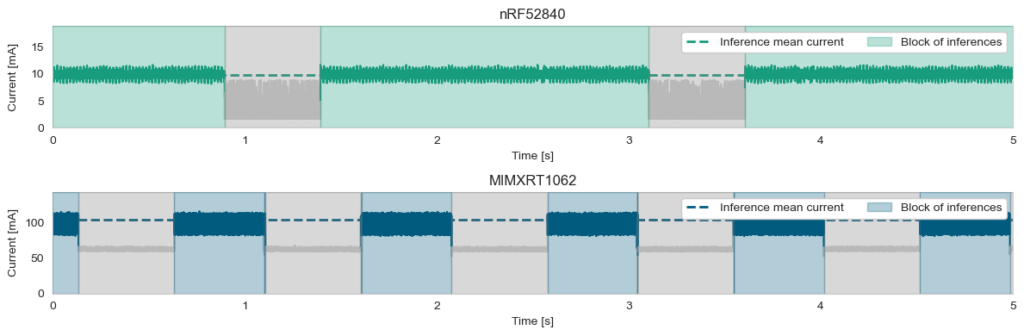
Microcontroller | Time per inference in ms | Energy per inference in µJ |
MIMXRT1062 | 0,47 | 160 |
nRF52840 | 17,0 | 555 |
Den Ergebnissen nach zu urteilen ist der MIMXRT1062 pro Inferenz effizienter als der nRF52840. Bisher wurde jedoch der Energiebedarf im Energiesparmodus außer Acht gelassen. In vielen Anwendungen ist es nicht notwendig, die Inferenz durchgängig mit einer hohen Frequenz durchzuführen, da sich z.B. der zu detektierender Zustand nur selten ändert oder eine schnelle Reaktionszeit nicht entscheidend ist. In diesem Fall kann man leicht argumentieren, dass eine Inferenz einmal pro Minute ausreichend ist. Das folgende Diagramm, zeigt die mittlere Leistungsaufnahme der Microcontroller in Abhängigkeit von der Inferenz Frequenz. Es ist ersichtlich, dass sich beide Microcontroller mit abnehmender Inferenz-Frequenz der Leistungsaufnahme in ihrem Energiesparmodus annähern und der Einfluss einer einzelnen Inferenz immer geringer wird. Bereits ab einer Inferenz alle 85ms arbeitet der nRF52840 effizienter als der MIMXRT1062.
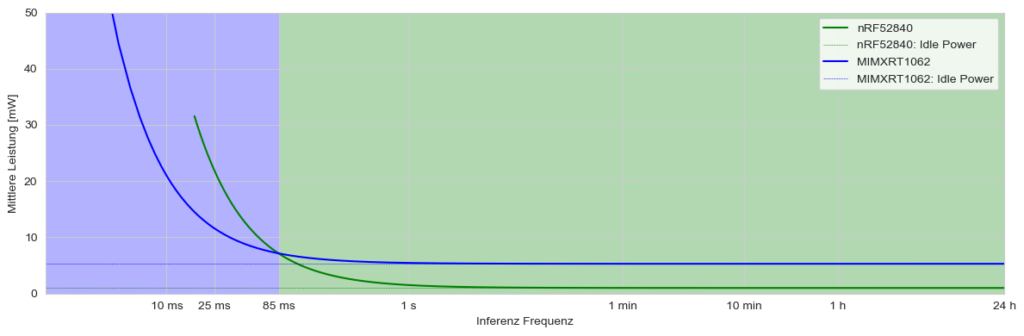
Mittlere Leistungsaufnahme in mW bei gegebener Inferenz Frequenz | ||||||||
Microcontroller | 10 ms | 25 ms | 85 ms | 1s | 1 min | 10min | 1 h | 24 h |
MIMXRT1062 | 20,97 | 11,56 | 7,13 | 5,45 | 5,30 | 5,29 | 5,29 | 5,29 |
nRF52840 | – | 21,78 | 7,11 | 1,51 | 1,00 | 0,99 | 0,99 | 0,99 |
Für eine Inferenz pro Minute benötigt der nRF52840 nur 1mW, während der MIMXRT1062 über 5mW benötigt. Dies bedeutet, dass das System bei gleicher Batteriekapazität mehr als fünfmal so lange läuft. Für diese Anwendung würde man sich daher eindeutig für den nRF52840 entscheiden. Über ein Jahr hinweg benötigt das System dann ca. 31 kJ. Mit zwei AA Alkaline Batterien würde das Retro-Fit System damit bereits ein Jahr lang auskommen. Der CO2-Fußabdruck des Systems beläuft sich somit auf etwa 416 g CO2eq/Jahr [3] was den CO2-Emissionen entspricht, die ein neues benzinbetriebenes Auto auf einer Strecke von ca. 4 km verursacht [4].
Allein in Deutschland wurden seit 2010 über 1,5 Mio. Wärmepumpen verkauft [5]. Angenommen man würde all diese Wärmepumpen mit einem solchen Retro-Fit Gerät ausstatten, so würden dadurch jährlich Emissionen in Höhe von 624 tC02eq verursacht. Dies entspricht dem CO2-Ausstoß von ca. 500 benzinbetriebenen Autos jährlich [4, 6]. Bei einem vergleichbaren System mit einem MIMXRT1062 würde entsprechend mehr als die fünffache Menge an CO2-Emissionen entstehen.
Fazit
Edge AI bietet eine vielversprechende Möglichkeit, nachhaltige Lösungen zu entwickeln, die nicht nur effizient, sondern auch umweltfreundlicher sind. Die Berücksichtigung des CO2-Fußabdrucks bei der Entwicklung von Edge AI-Anwendungen kann dazu beitragen, die Auswirkungen auf die Umwelt zu reduzieren und gleichzeitig die Lebensdauer der Systeme und damit den Nutzen der Anwendung zu erhöhen.
Quellen:
[1] Abadade, Y., Temouden, A., Bamoumen, H., Benamar, N., Chtouki, Y., & Hafid, A. S. (2023). A comprehensive survey on tinyml. IEEE Access. [2] Sandbag (Ember). (31. Januar, 2024). Entwicklung des Emissionsfaktors der Stromerzeugung in Deutschland und Frankreich im Zeitraum 2000 bis 2023 (in g CO2-Äquivalent pro Kilowattstunde Strom) [Graph]. In Statista. Zugriff am 26. September 2024, von https://de.statista.com/statistik/daten/studie/1421117/umfrage/emissionen-strom-deutschland-und-frankreich/ [3] Benecke, S. (2019). Systemverhalten von Energy Harvestern in autonomen Sensoren unter Betrachtung der Wechselwirkung von Funktionalität und Umweltverträglichkeit (Doctoral dissertation, Technische Universität Berlin). https://www.deutsche-digitale-bibliothek.de/item/I75CKRUQ46CTYBJBMIE5PXFZHC2OKH2E [4] JATO. (21. April, 2021). Durchschnittlicher CO2-Ausstoß der in Europa neu zugelassenen Pkw nach Antriebsarten von 2017 bis 2020 (in Gramm pro Kilometer) [Graph]. In Statista. Zugriff am 26. September 2024, von https://de.statista.com/statistik/daten/studie/985204/umfrage/durchschnittlicher-co2-ausstoss-von-pkw-nach-antriebsarten-in-europa/ [5] Bundesverband Wärmepumpe. (22. Januar, 2024). Absatz von Heizungswärmepumpen in Deutschland in den Jahren 2010 bis 2023 (in 1.000) [Graph]. In Statista. Zugriff am 26. September 2024, von https://de.statista.com/statistik/daten/studie/217750/umfrage/absatz-von-heizungswaermepumpen-in-deutschland/ [6] Kraftfahrt Bundesamt. (Juni 2021). Kurzbericht Inländerfahrleistung. Zugriff am 26. September 2024, von https://www.kba.de/DE/Statistik/Kraftverkehr/VerkehrKilometer/vk_inlaenderfahrleistung/2020/2020_vk_kurzbericht.html